You signed in with another tab or window. Reload to refresh your session.You signed out in another tab or window. Reload to refresh your session.You switched accounts on another tab or window. Reload to refresh your session.Dismiss alert
I`m new in this field and not good at the mathematics.
Recently I`m doing two sample MR analysis between metabolome sumstats by using MRlap. I found most of my results have extremely large corrected_effect_se value (corrected_effect_p is nearly 1) while the observed_effect_se seems usual (observed_effect_p is very low and significant) and p_difference is nearly 1 (as below). May I ask how to interpret the results and which value (corrected or observed) should I use in this case.
For the GWAS sumstats, both of them are analyzed by about 10000 samples, and sample overlap rate is 100%, number of IVs is 14 to 22. I also attached my R code below. This is a common issue and I also found the similar case by using other sumstats (n=100,000, sample overlap rate 10%).
The text was updated successfully, but these errors were encountered:
Hello,
Sorry for not being able to reply before.
It would have been helpful to see the log, not just the function call and the results. As described in the paper, the heritability and the polygenicity are very important parameters to estimate the corrected effect, and the variance of the corrected causal effects is estimated through a sampling strategy (the number of simulations needed to estimate the variance would help detect abnormal situations), which could fail if the heritability is too low or if the trait is not polygenic enough. Could this be the case with metabolomics data?
Hi,
I`m new in this field and not good at the mathematics.
Recently I`m doing two sample MR analysis between metabolome sumstats by using MRlap. I found most of my results have extremely large corrected_effect_se value (corrected_effect_p is nearly 1) while the observed_effect_se seems usual (observed_effect_p is very low and significant) and p_difference is nearly 1 (as below). May I ask how to interpret the results and which value (corrected or observed) should I use in this case.
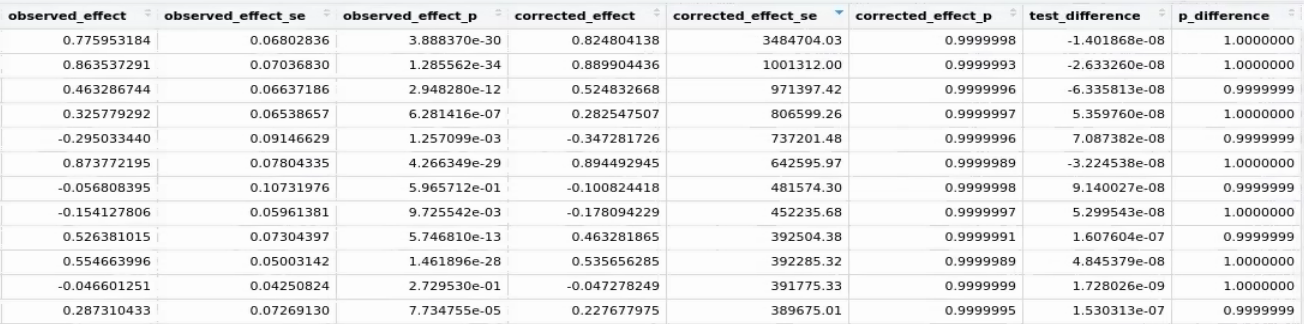
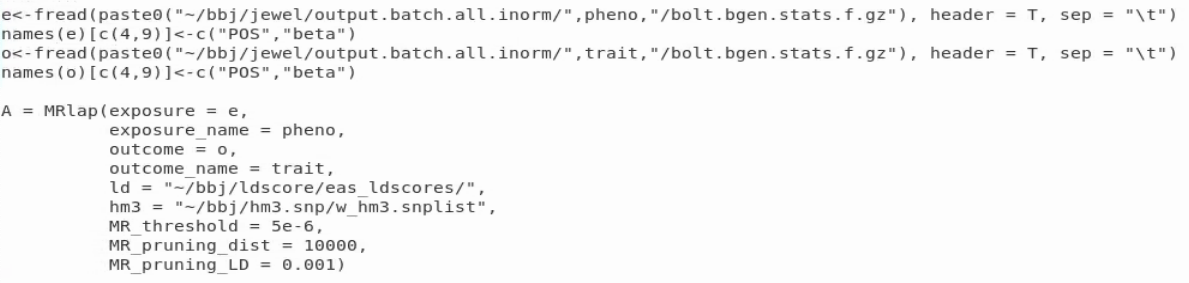
For the GWAS sumstats, both of them are analyzed by about 10000 samples, and sample overlap rate is 100%, number of IVs is 14 to 22. I also attached my R code below. This is a common issue and I also found the similar case by using other sumstats (n=100,000, sample overlap rate 10%).
The text was updated successfully, but these errors were encountered: